Experimentation Works: The Surprising Power of Business Experiments
Stefan Thomke, an authority on the management of innovation, is the William Barclay Harding Professor of Business Administration at Harvard Business School. He has worked with global firms on product, process, and technology development, customer experience design, operational improvement, organizational change, and innovation strategy. Professor Thomke has been pioneering in the field of experimentation since before
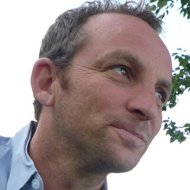
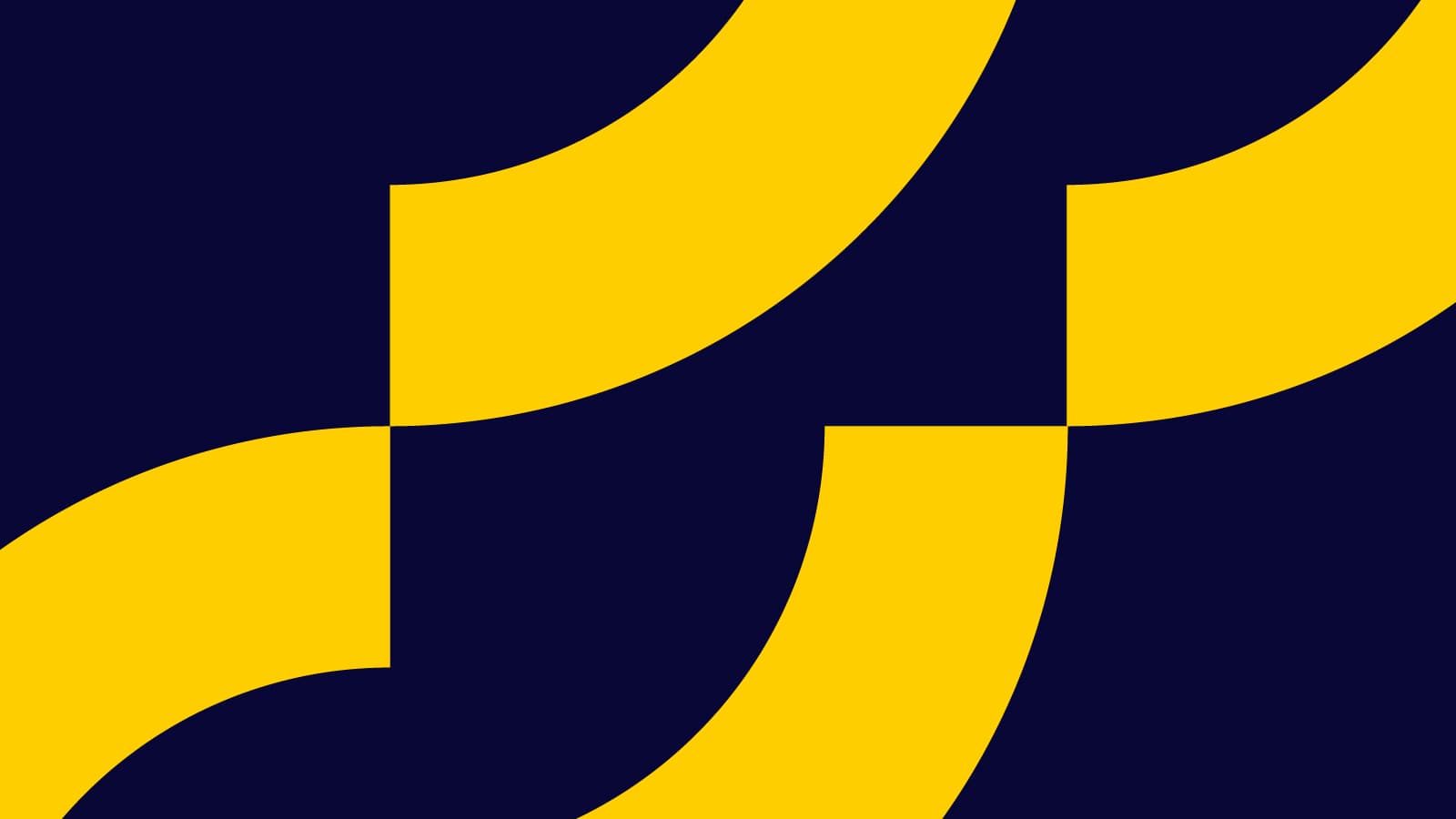
Stefan Thomke, an authority on the management of innovation, is the William Barclay Harding Professor of Business Administration at Harvard Business School. He has worked with global firms on product, process, and technology development, customer experience design, operational improvement, organizational change, and innovation strategy. Professor Thomke has been pioneering in the field of experimentation since before Optimizely was founded, with his first book on the topic, ‘Experimentation Matters: Unlocking the Potential of New Technologies for Innovation‘ coming out in 2003.
His new book ‘Experimentation Works: The Surprising Power of Business Experiments‘ was recently published and in addition to collaborating with him, we had the chance to sit down with him and discuss some of his findings.
Optimizely: Why did you write ‘Experimentation Works?’
Stefan Thomke: When I published my first book “Experimentation Matters: Unlocking the Potential of New Technologies of Innovation” in 2003, I made a prediction: digital experimentation tools not only had the potential to revolutionize a company’s R&D, but they could also transform entire industries by shifting experimentation—and thus innovation—to users and customers.
Five years later, Apple opened its App Store, which empowered anyone, anywhere to design and distribute novel applications. By early 2017, about 2.2 million apps had become available to iOS users. And, as anyone who closely follows simulation and prototyping tools knows, their use has become pervasive in manufacturing businesses, even though companies still grapple with the integration and management issues I wrote about in 2003. As I happily watched these predictions come true, I thought that it was time to move on and study another topic. And I was wrong!
Here’s why: In 2003, Google had just completed year five, Amazon was nine years old, and Booking.com was still an independent startup in Amsterdam. Even though I had studied the statistical and management principles that are core to experimentation, I had not closely examined their role in customer experience and business model design. I had no idea how their use would fuel the rise of today’s online businesses. When it finally came to my attention, I realized right away that large-scale, controlled experimentation would revolutionize the way all companies operate their businesses and how managers make decisions.
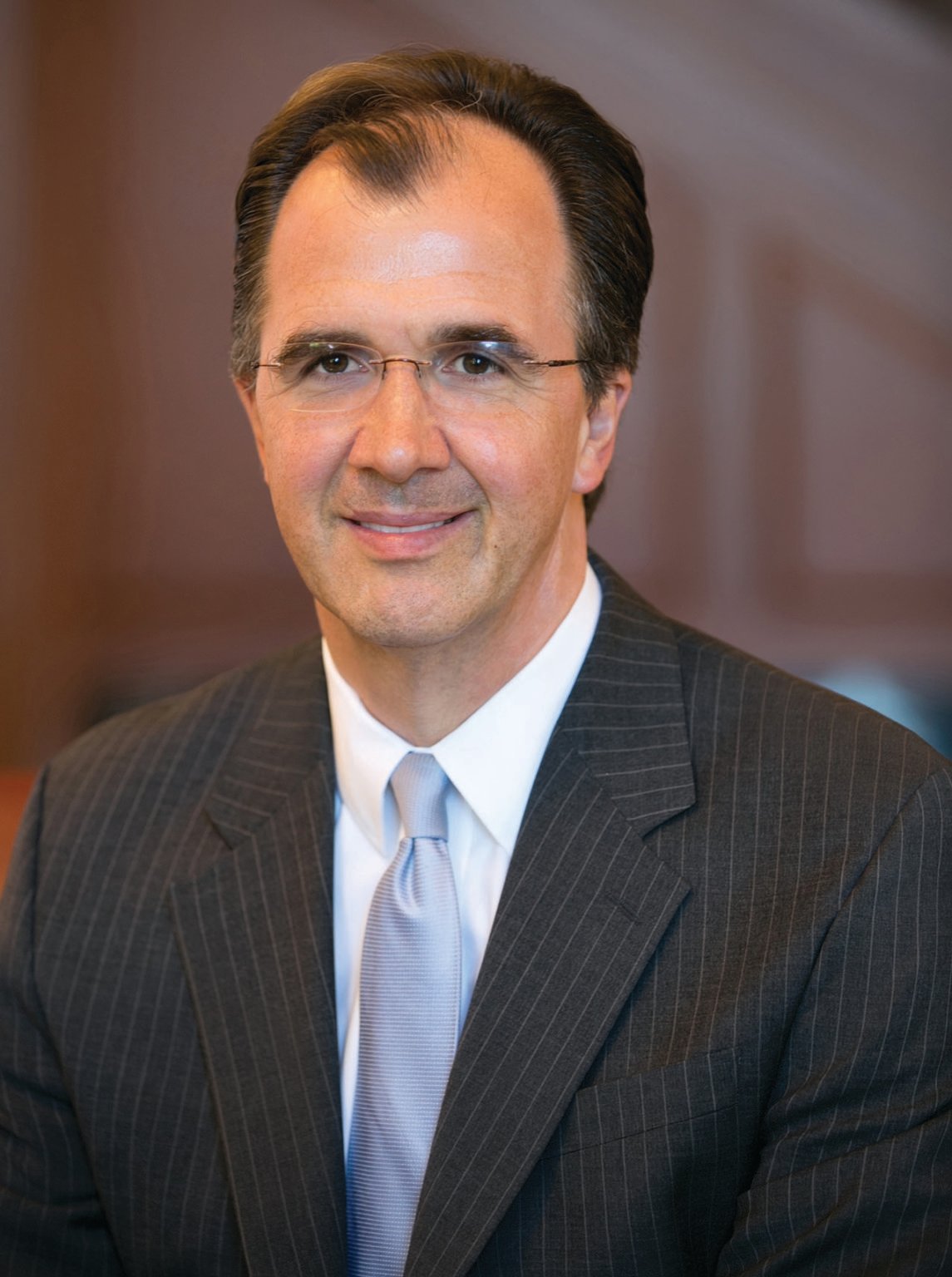
Harvard Business School Professor Stefan Thomke
Optimizely: In the preface you mention that many readers think large-scale business experimentation affects only businesses with digital roots and you share how there are three reasons that you think this book will change their mind, would you share with us those three reasons?
Thomke: For those readers who think that large-scale business experimentation affects only businesses with digital roots, I hope that my new book will change their mind—for three reasons.
First, companies without digital roots are increasingly interacting with customers online. Taking advantage of the huge number of digital touch points, design choices, and business decisions is simply overwhelming without access to large-scale testing.
Second, the ideas and principles covered in the book are applicable to any business setting, whether you’re offline or online, B2C or B2B, in manufacturing, retail, business and financial services, logistics, travel, media, entertainment, healthcare, and so on.
Third, companies without software roots should abide by venture capitalist Marc Andreessen’s maxim, “Software is eating the world.” I’ve seen many hardware development projects where software ate more than half of all resources. Consider that best software development practices have changed dramatically in the last decade. At Microsoft’s Bing, about 80 percent of proposed changes are first run as controlled experiments.
Optimizely: Optimizely shared data with you and Sourobh Ghosh – what were the preliminary findings?
Thomke: To understand how organizations are testing business hypotheses, Optimizely gave us access to all experiments, as anonymized data, that its customers ran from November 2016 to September 2018. Using this data, we created a large database that was carefully checked for robustness and data integrity. Experiments were filtered along several quality criteria, such as sufficient customer traffic (more than one thousand visitors per week), true experiments (no A/A tests or bug fixes), and so on.
Here is what our preliminary analysis found:
- The average number of variations, in addition to a control, was 1.5 (median was 2) and about 70 percent of experiments were simple A/B tests. It’s not clear if organizations deliberately kept tests simple or just started out that way.
- The median duration of an experiment is 3 weeks but the average was 4.4. Here is why. Many experiments just “lingered” for months and it’s hard to justify why some tests should run beyond fifteen or twenty weeks. Most likely, it’s an indication of poor organizational practices and a lack of process standards.
- The industry segments that experimented the most in our study were retail, high-tech, financial services, and media. We found that high-tech companies are the most “efficient” testers (greater lifts per experiment).
- Overall 19.6 percent of all experiments achieved statistical significance on their primary metric. Here is a caveat: 10.3 percent had positive and 9.8 percent had negative significance. If the primary metric is (positive) customer conversion, a negative result could stop companies rolling out features that create losses, assuming it holds up in future experiments.
- The large dataset also allowed us to answer a fundamental question: Do variations perform better than the baseline? To be sure, we removed outliers so the analysis wouldn’t be skewed and ended up with more than thirty thousand variations. The evidence strongly suggested that, on average, variations did better than the baseline (p = 0.000). In other words, a resounding yes that experimentation works!
Optimizely: What are important developments that will require massive experimentation capacity?
Thomke: Here are three important developments that will require massive experimentation capacity.
First, customers will increasingly interact with your company through mobile devices (smartphones, tablets, watches, etc.). In 2018, companies shipped more than 1.5 billion smartphones and mobile devices; units shipped are expected to exceed 2 billion by 2023. But what’s more amazing is the computational and networking power of these devices. If the rate of progress continues, customers will have today’s supercomputers (used by researchers to forecast global weather patterns or to simulate the early moments of the universe) in their pockets a few decades from now. This will result in an explosion of touch points and complex interactions with customers, including behaviors and value drivers that we’re not even aware of today. These new customer experiences that will need a lot of exploring and optimizing. The only way for all companies to keep up with these rapid developments and adjudicate what does and does not work is by running large-scale experimentation programs.
Second, companies will soon recognize that a business analytics program is incomplete without controlled experiments. Traditional analytics using big data looks in the rearview mirror and, for innovation, suffers from serious limitations: the greater the novelty of an innovation, the less likely it is that reliable data will be available. (In fact, if reliable data had been available, someone would have already launched the innovation and it wouldn’t be novel!)
Finally, the third, and perhaps the most significant, development that will require massive experimentation capacity is the rise of artificial intelligence (AI)—or more specifically, machine learning and artificial neural networks. Sophisticated algorithms and biology-inspired neural networks can be trained with large datasets to detect patterns with a high degree of automation (e.g., identification, clustering, and prioritization of user problems). Even though most of the theoretical breakthroughs were made decades ago, we’re finally witnessing an explosion of applications that will change the future of businesses. Imagine the following: What if AI-based methods could analyze your data (customer support information, market research, and so on) and generate thousands of evidence-based hypotheses? Now imagine that these algorithms could also design, run, and analyze experiments with no management involvement at all. Large-scale experimentation programs using a closed-loop system can run in the background and make recommendations for action when you come to work in the morning. And you can have a high degree of confidence that your actions will produce results because they were scientifically tested for cause and effect.