From ideation to results: AI experimentation is changing how we run tests (for real)
AI is improving experimentation—generating test ideas, automating variations, and analysis for faster, smarter results. Learn how AI can optimize your A/B testing strategy.

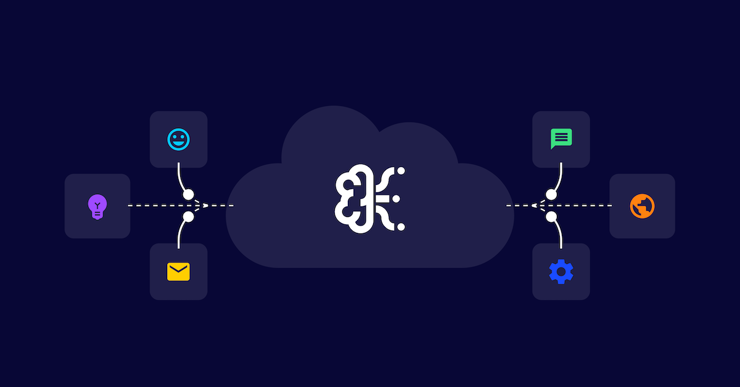
What if you could run more experiments in half the time without spreadsheets, dev bottlenecks, or statistical confusion?
AI-powered experimentation is making this a reality today.
Here's everything you need to know about how AI is improving experimentation, including what's already possible and steps you can take right now to deploy AI in your experimentation program.
AI vs. traditional A/B testing: The complete experimentation workflow
Here’s how the current experimentation process (beyond A/B testing) looks:
Image source: Optimizely
With AI in the frame, the fundamentals of experimentation aren't changing, but AI is removing the roadblocks that typically slow teams down.
Here’s how:
Experimentation challenges | AI-powered experimentation |
Racking your brain for test ideas? | AI suggests automated A/B test ideas. Plus, targeted experiments based on your data and goals |
Not sure about your test setup? (Math is hard) | AI guides you through selecting the right metrics, audience size, and duration for confident results |
Not sure which metric will get you to statistical significance in enough time? | AI helps you understand what metrics to select to get to results, fast |
The dev team is backed up (Translation: See you in 3 months) | AI automates implementation, reducing technical dependencies |
Are data and those graphs giving you a headache? | Get clear, actionable insights with AI-powered analysis |
Can't connect test results to business outcomes? (not sure what to do next) | Receive data-driven recommendations for follow-up tests |
The destination hasn't changed, but the ride will get a lot smoother with AI.
How does AI make it easier to run more quality experiments?
Before AI: Manual test ideation, slow development cycles, and complex analysis meant teams constantly hit resource walls. Whether it is waiting for developers, lacking dedicated experimentation specialists, or not having enough time to properly analyze results, teams struggle to maintain momentum. Even with the right tools, running a quality experimentation program required significant investment in people and time that many organizations couldn't sustain.
With AI: AI-powered optimization for experiments improves the entire workflow
Image source: Optimizely
1. Faster variation creation
AI variation generator pre-builds multiple variations
Today, AI just doesn't suggest one-off changes during variation creation, it works upstream in the process, recommending complete experiments with multiple variations built-in. When AI suggests an experiment, it includes the variations you should test.
Coming soon:
- AI will analyze screenshots or URLs automatically
- Provide specific test suggestions in the chat window
- Have ideas waiting for you when you log in
- AI will even pre-create the code changes for you
2. Smarter metric selection
AI helps avoid long test times
Two approaches here: Guidance and Suggestion.
For more advanced users, there will be guidance. For example, if you pick a metric that'll take a long time to reach statistical significance, AI will let you know and offer other suggestions.
For newer users, AI will jump right into suggesting a test plan with ready-to-go metrics (and let's be real, we all could use a helping hand now and then) so they can start testing with confidence.
Key factors AI considers when choosing experimentation metrics:
- Relevance: How closely the metric connects to what you're testing.
- Statistical power: Whether you'll get enough traffic and conversions to reach significance in a reasonable timeframe.
- Business alignment: How well the metric ties to what your business and industry truly care about.
3. Reduce dev bottlenecks
AI handles implementation
Our analysis of 127,000 experiments showed teams achieved the highest impact with less than 10 tests per engineer. AI can help you achieve this sweet spot even if you're resource-limited.
By letting AI handle routine implementation tasks, you can maintain that sweet spot of developer productivity while scaling your program. It suggests templates, implement them, and write the code so your team can run more experiments without constantly tapping your development resources.
4. Interpreting results
Helping you and your business make decisions, faster
For teams who find data analysis challenging, AI turns complex test results into simple insights. Instead of wrestling with statistical significance and confidence intervals, your AI partner:
- Translates data into clear takeaways ("Variant B increased conversions by 15%")
- Highlights what worked best ("The simplified form drove the biggest impact")
- Suggests next steps ("Test this form design on other landing pages")
- Spots hidden patterns ("This worked especially well for mobile users")
The result? Everyone on your team can understand outcomes and make data-driven decisions confidently, regardless of their statistical expertise.
The science behind AI-powered experimentation
We know AI-washing is real, and the skepticism around AI in enterprise software is valid. Security concerns, trust issues, and data governance are legitimate challenges that need careful consideration.
At Optimizely, we're taking a measured, research-based approach. Rather than jumping on the AI bandwagon, we're asking ourselves the hard questions:
- How does AI-generated test ideation actually compare to recommendations from seasoned experimentation teams?
- Beyond the surface appeal, what tangible value do these AI features bring to mature experimentation programs?
- How do we ensure AI enhances rather than replaces human expertise in experimentation?
Our methodology is grounded in Human Centered Design. We started by mapping real-world experimentation processes, identifying genuine friction points, and designing solutions that complement, not disrupt existing workflows.
This means studying how experimentation teams work, understanding their concerns about AI integration, and building features that address real needs while maintaining data security and user trust. The goal isn't to add AI for AI's sake but to thoughtfully augment human capabilities where it makes the most sense.
What is our overall AI strategy?
We want to help experimenters run an experiment from ideation to interpretation and then help them do it again.
In 2025, we're building an experiment creation agent to solve challenges for four steps of the experimentation lifecycle: Analyze/Ideate, Design, Development, and Interpret.
Step: Experiment ideation
Customers can get started right away with individual ideas, generate hypothesis or read a report the agent generates.
Image source: Optimizely
Step: Test plan
Opal will set up an initial test plan complete with a hypothesis and metric. Users will also get sources for concepts like minimal detectable effect.
Image source: Optimizely
Step: Development
Customers will get ready-made blocks to implement their experiments.
Image source: Optimizely
Step: Interpretation and results
You can quickly understand the results of your tests and the "So what?"
Image source: Optimizely
However, at least today/near term, AI isn't going to remove the need to do some validation/research on your own.
AI can suggest experiment and a/b testing ideas, but if it doesn't have access to your product's analytics, it will be limited in what it can do. The best ideas will still be grounded in actual analytical data you have that point to an issue to address.
For now, AI is here to help you ideate, refine, and become better at the craft. But it can't run the experimentation lifecycle entirely for you.
But does it even work for my industry...
We're still in the early stages of industry-specific AI features. While some industries like banking and insurance face more regulatory hurdles than software and retail, AI is getting smarter about different industry needs.
For instance, the website analyzer already applies industry-specific principles when suggesting experiment ideas. Soon, you'll see even more tailored guidance for your specific sector.
Take metrics selection, for example. We know that education companies care deeply about CTA clicks, while consumer goods businesses focus on checkout metrics. AI will help you prioritize what matters most in your industry, right from the start.
Looking ahead, we're working on making AI even more industry-aware. For example:
- Banking: Secure experiments that keep compliance in check.
- Retail: AI-driven tests that adapt to changing shopper behavior.
- Software: Code-driven testing that builds user trust.
- Insurance: AI simplifies complex decision-making.
Getting starting with AI experimentation
You don't need a special "AI adoption roadmap" to get started with AI experimentation. The experimentation process stays the same, AI just makes each step easier and more efficient.
Instead of learning a whole new way of working, you'll find that familiar tasks just flow better. Whether you're a marketing team looking to optimize campaigns or a product team focused on user experience, AI reduces friction at every step of your existing process.
For example, we started by looking at where teams get stuck - like coming up with test ideas. It was a natural place to begin because we saw that not running enough experiments was directly impacting user retention. By letting AI tackle this friction point first, teams could move forward more easily.
And here's an interesting shift we're seeing: while many teams started out AI-skeptical (totally normal!), that hesitation is quickly fading as they see real results. Over the next 12 months, we expect this skepticism to transform into enthusiasm as AI proves itself as a reliable partner in the experimentation process.
The bottom line? Just start experimenting. Experimental AI is here to help, not to complicate things.
Experimentation agents: Can AI make it rain harder in the future?
The next step is capable AI agents. Agents introduce some autonomy to the process, so instead of having to go and prompt every time, the AI tool can anticipate needs and go do work autonomously.
Imagine logging into your testing platform and finding:
- Test ideas already generated and waiting for you
- Multiple variations with code ready to go
- Proactive suggestions based on your goals
Think of it as upgrading from an AI assistant to an AI partner. While current AI helps when you ask, AI agents will be working behind the scenes, spotting opportunities and doing the groundwork before you even think to ask.
For example, imagine you're a product manager running pricing experiments. Instead of starting from scratch, you arrive Monday morning to find your AI partner has already:
- Analyzed weekend traffic patterns and spotted an opportunity to test your premium tier pricing
- Generated three test variations based on successful pricing models in your industry
- Drafted a test plan with power calculations and target segments
- Flagged potential risks based on previous pricing tests
All this proactive groundwork means you can focus on strategic decisions rather than starting with a blank slate. And since the AI has learned from your historical experiments, its suggestions are tailored to what works for your specific audience.
AI in experimentation isn't some far-off dream. We're talking about capabilities that could be here as soon as the next three to six months.
Wrapping up
The potential of AI in experimentation is clear through accelerated workflows, amplified creativity, and more time for strategic thinking. But what excites us most at Optimizely isn't just AI assistance, it's the evolution toward true AI partnership.
We're building toward a future where AI agents work proactively across your entire marketing and experimentation ecosystem, from surfacing test opportunities to ensuring brand compliance and connecting cross-product insights.
Whether you're personalizing customer experiences in retail or optimizing feature rollouts in software, AI-powered experimentation gives you the competitive edge to lead the change.