Bayesian vs Frequentist statistics
Just like a suspension and arch bridges both successfully get cars across a gap, both Bayesian and Frequentist statistical methods provide to an answer to the question: which variation performed best in an A/B test?

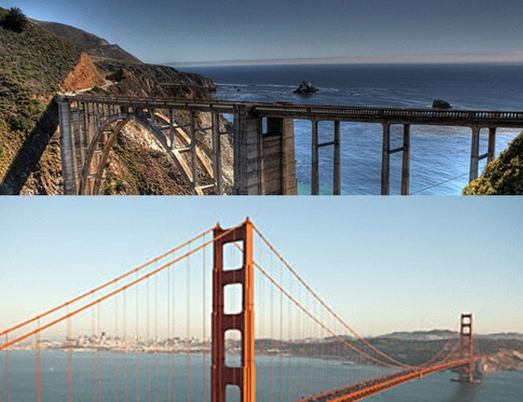
Statistics are an essential component of understanding your A/B test results—methods of computing a single number that determines whether you can take action on implementing a variation over the experiment control. However, there are many ways to arrive at that number. Which method should you use?
Two commonly referenced methods of computing statistical significance are Frequentist and Bayesian statistics. Historically, industry solutions to A/B testing have tended to be Frequentist. However, Bayesian methods offer an intriguing method of calculating experiment results in a completely different manner than Frequentist. In the world of statistics, there are devotees of both methods—a bit like choosing a political party.
In January, we released Stats Engine and took a moderate stance: You should be able to take advantage of Bayesian elements in your results, and use them to support Frequentist principles that provide stability and mathematical guarantees.
In this post, we’ll cover the benefits and shortcomings of each method, and why Optimizely has chosen to incorporate elements of both into our Stats Engine.
What are Bayesian and Frequentist Statistics?
Bayesian statistics take a more bottom-up approach to data analysis. This means that past knowledge of similar experiments is encoded into a statistical device known as a prior, and this prior is combined with current experiment data to make a conclusion on the test at hand.
On the other hand, Frequentist statistics make predictions on underlying truths of the experiment using only data from the current experiment. Frequentist arguments are more counter-factual in nature, and resemble the type of logic that lawyers use in court. Most of us learn frequentist statistics in entry-level statistics courses. A t-test, where we ask, “Is this variation different from the control?” is a basic building block of this approach.
The goal of an A/B test, statistically speaking, is to determine whether the data collected during the experiment can conclude that one variation on a website or app is measurably different from the other. Bayesian and Frequentist approaches will examine the same experiment data from differing points of view. Like a suspension versus arch bridge above, they strive to accomplish the same goal. Both structures serve the purpose of crossing a gap, and in the case of A/B testing, both Bayesian and Frequentist methods use experiment data to answer the same question: which variation is best?
What are the benefits of either approach?
A/B testing platforms like Optimizely use Frequentist methods to calculate statistical significance because they reliably offer mathematical ‘guarantees’ about future performance: statistical outputs from an experiment that predict whether or not a variation will actually be better than the baseline when implemented, given enough time. For instance, with Frequentist guarantees, we can make statements like: “Fewer than 5% of implemented variations will see improvements outside their 95% confidence interval.”
For more knowledge on this topic, download the eBook, A Practical Guide to Statistics for Online Experiments.
Bayesian tests, on the other hand, make use of prior knowledge to calculate experiment results. The biggest advantage of Bayesian approaches is that they put to use the prior knowledge each experimenter brings to the table. Using all the information at your disposal, whether current or prior, should lead to the quickest possible experiment progress. Provided that the assumptions made using historical data to calculate the statistical prior are correct, this should help experimenters to reach statistically significant conclusions faster.
However, Bayesian methods do not always come with the same guarantees as Frequentist methods about future performance. If we were to automatically use them as if they did, applying Frequentist sentences—like the above one for confidence intervals—to Bayesian calculations, we could be led to an incorrect conclusion. This is because of the risk that prior experiment knowledge may not actually match how an effect is being generated in a new experiment, and it’s possible to be led astray if you do not account for it.
In a New York Times article from last year describing applications of Bayesian statistics, the author considers an example of searching for a missing fisherman. The Coast Guard was able to use data about local geography and past searches in combination to make predictions about which areas were more likely contain their missing fisherman. As more information on the current search surfaced, these inputs were combined with knowledge of nature’s prior behavior to accelerate the search, which resulted in a happy ending.
The main pitfall in extrapolating this success story to A/B testing is that incorporating prior beliefs that don’t match with reality can have exactly the opposite effect—an incorrect conclusion and a slower path to the right answer. A purpose of A/B Testing is to learn from your experiment to make future actions, whether it’s to implement a variation, or run more tests. The prior information you have today may not be equally applicable in the future.
Test + Learn: Experimentation
This is effectively like using a map from a maze that you previously completed to navigate a new one. It could help you complete the maze faster, or it could lead you down the wrong path, taking longer to find the exit.
Ultimately, misunderstanding or misuse of statistics will give poor results no matter what kind of statistical method is applied (Bayesian or Frequentist.) It is for this reason that strong fundamentals are critical for good A/B testing, and why we prioritize incorporating a robust version of these statistics into our product. Solid statistical statements, and presenting them in an accessible way, is a greater benefit to our customers than squeezing out every last drop of efficiency.
What does the future look like for Frequentist and Bayesian advocates?
Yet as we developed a statistical model that would more accurately match how Optimizely’s customers use their experiment results to make decisions (Stats Engine), it became clear that the best solution would need to blend elements of both Frequentist and Bayesian methods to deliver both the reliability of Frequentist statistics and the speed and agility of Bayesian ones.
This approach is along the lines of a somewhat less well known third school of thought in statistics. It is called Empirical Bayes and is based on the principle that statistical methods should incorporate the strengths of both Bayesian and Frequentist ideologies, while mitigating the weaknesses of either.
Like the bridge concept, Empirical Bayes combines both approaches to provide an innovative solution to the questions at hand, and can help to avoid the difficulties of choosing either an arch or suspension bridge alone.
Combining the best of an arch and suspension construction creates a through arch bridge, which can provide the best outcome for a given gap, as seen here with the Sydney Harbor Bridge.
In fact, Optimizely’s Stats Engine incorporates a method directly from the Empirical Bayes line of thinking, so that users can test many goal and variation combinations without sacrificing statistical accuracy.
The Benjamini-Hochberg approach controls a type of statistical error called False Discovery Rates (FDR.) FDR is a measurement that addresses the fact that you can make many errors when running multiple A/B tests simultaneously. This is typically a problem if you run multivariate or A/B/n experiments with many variations, or track many goals in an experiment.
We detail how this approach works and why it presents the statistical error rate that businesses actually care about in our blog post on Stats Engine and more detailed technical writeup. We have also recently recorded a webinar with an example of FDR in action for A/B Testing.
The Benjamini-Hochberg FDR approach for controlling this error has proven to be successful by both Frequentist and Bayesian standards. The procedure not only reasonably incorporates prior experiment data, but also gives the results and Frequentist statistical guarantees you would expect, no matter which perspective you take.
The rapid and far-reaching acceptance of the Benjamini-Hochberg approach in academic and medical environments can be attributed to the fact that the method has convinced both Bayesians and Frequentists of its merits.
So do we think that everyone should think like a Frequentist? A Bayesian? An Empirical Bayesian? Not at all. Should you hurry to take up the colors of one of these camps? Of course not. The reason these ideologies persist is that at a really basic level they are all good ways to think about learning from your data.
We feel that in order to be a knowledgeable A/B Tester, like an informed voter, or an effective structural engineer, it is important to be knowledgeable of the choices available to you. We’re excited about not only finding the best statistics to fit the way you use data to make decisions and take action, but also empowering you to use them.