Warehouse-native analytics: The future of data-driven experimentation
Traditional experimentation only tells half the story. See how warehouse-native analytics enable organizations to test against true business outcomes, analyze cross-channel data, and make faster, more informed decisions, all while keeping entire data in-house and maintaining governance.

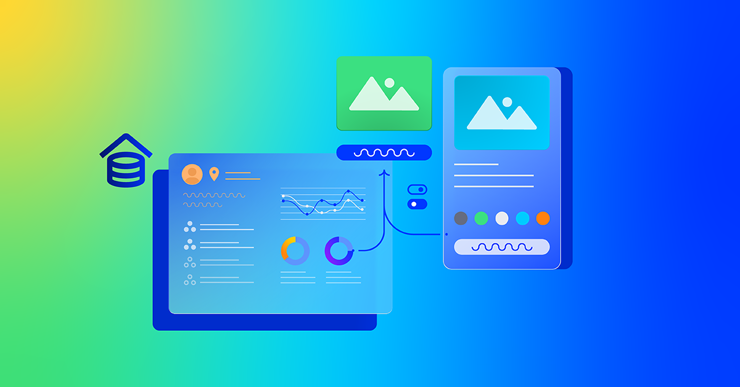
Traditional experimentation platforms promise data-driven decisions, but they're continuously falling short where it matters most.
While teams can track surface metrics like page views and click rates, they can't answer crucial questions about return rates, revenue impact, or customer lifetime value without moving sensitive data out of their warehouses.
Want to understand how your experiments affect customer lifetime value (CLV) or return rates? That requires moving sensitive data across systems or building complex data pipelines.
But the problems run deeper than just disconnected data:
- Teams struggle with data silos that limit their view of customer behavior.
- They waste resources duplicating data across platforms, creating security risks and governance challenges.
- Most critically, they can't experiment with their most important business metrics because that data never leaves their warehouse.
"This fundamental shift in how organizations manage and utilize their data demands a new approach," explains Vijay Ganesh, founder and CEO of NetSpring. "Companies need to bring analytics to where their data lives, not the other way around."
Vijay Ganesan, VP, Software Engineering, discusses what warehouse-native analytics actually means.
Understanding warehouse-native analytics
Warehouse-native analytics fundamentally changes how teams measure success. By connecting directly to your data warehouse, teams can finally test against the metrics that actually influence business outcomes. This approach focuses on five core elements to help experimentation teams:
- Business outcome attribution: Data teams can stop building complex data pipelines just to understand experiment results. Test directly against metrics already in your warehouse, from revenue and return rates to customer lifetime value. Want to know if your new feature drives subscription renewals? That insight is now at your fingertips.
- On-the-fly explorations: Data teams no longer need to write complex queries for every analysis. They can generate cohort-specific insights on the fly, dramatically reducing the time from question to insight. Want to know how high-value customers from specific regions respond to your latest test? That analysis happens instantly.
- Warehouse-native stats: Your customer data doesn't live in silos. Why should your experiments? Run tests across all your digital channels by leveraging warehouse data through Optimizely's Stats Engine. Email campaigns, CRM metrics, web behavior - analyze it all in one place, understanding true cross-channel impact.
- Safety, security, and compliance: Keep your sensitive data exactly where it belongs - in your warehouse. No more compromising between innovation and compliance. Financial institutions can now run sophisticated experiments while maintaining complete control over their data location and access.
- Data consistency: End the endless debates about whose numbers are right. When everyone works from the same warehouse data, you eliminate discrepancies between experimentation and analytics platforms. One source of truth means teams can focus on insights, not reconciling reports.
Shafqat Islam, President of Optimizely, discusses how warehouse-native analytics impact business data.
Benefits of warehouse-native analytics
Here's how warehouse-native analytics improves testing and decision-making processes for different teams:
Measuring true business impact
A major retailer wanted to understand how checkout page optimizations affected their bottom line. "Traditional testing would only show immediate conversion changes," explains Vijay. "But with warehouse-native analytics, they discovered their winning variation not only improved checkout completions but also reduced return rates by 20% - driving significant profit improvements."
Advanced analysis without the wait
Want to understand how different customer segments respond to your experiments? Warehouse-native analytics turns complex analysis into instant insights. Drill down into specific cohorts, visualize customer journeys, and spot trends that would have taken days to uncover with traditional methods.
Test + Learn: Experimentation
Cross-channel visibility
Customer journeys don't happen in a vacuum. A customer might see an email, visit your website, and complete a purchase through your app. Warehouse-native analytics connects these dots, showing you how experiments impact behavior across all channels.
Future-proof your experimentation
As your testing program grows, so do your analytics needs. Warehouse-native analytics scales with you:
- Run more sophisticated tests without performance penalties
- Access historical data for deeper insights
- Connect new data sources without rebuilding infrastructure
Vijay Ganesan, the CEO of NetSpring, discusses the impact of analytics on revenue
Warehouse-native analytics implementation strategy
You’re ready for warehouse-native analytics if you’re:
- Already incorporating a data warehouse into your data infrastructure
- Running experimentation results out of your experimentation platform
- Want to analyze experimentation results against business metrics
The transition to warehouse-native analytics is straightforward with out-of-the-box support for BigQuery, Snowflake, Amazon Redshift, Databricks, and Presto.
Three key stages:
And warehouse-native analytics isn't just about connecting to your data, it's about making that data work for you. For example:
- Smart sampling delivers quick results for ad-hoc explorations
- Auto-materialization identifies and optimizes frequent query patterns
- Specialized query optimization for time-series analysis
- The system is built to handle millions of events efficiently
These capabilities ensure teams can explore data freely without worrying about performance constraints or processing limits.
Wrapping up...
By eliminating data silos and enabling direct analysis within your data warehouse, teams can make faster, more informed decisions while maintaining data governance and reducing operational complexity.
The ability to combine experimentation with your consolidated customer data opens new possibilities:
- Run experiments using your complete customer data
- Make decisions based on true business outcomes
- Scale experimentation across products and features
- Measure impact through controlled tests that matter
Ready to test against your most important business metrics? See warehouse-native analytics in action.