Want better AI content? Your marketing team needs RAG
RAG is how AI agents reference your brand's knowledge base. Unlike standard AI which relies on generic, limited training data, RAG-powered AI pulls from your actual brand guidelines, product listing details, content libraries, and customer behavior data.

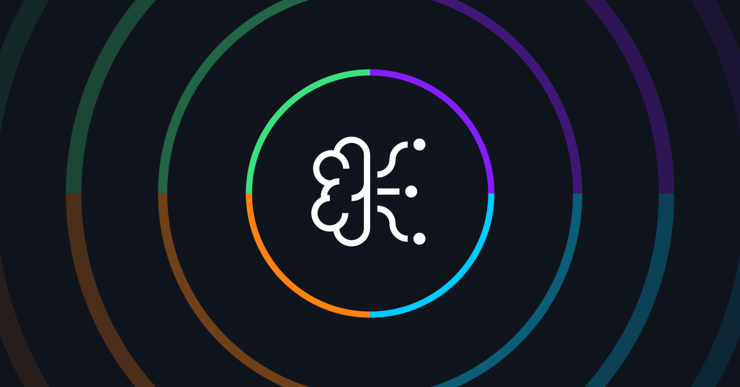
Six revisions deep into an AI-generated blog post and it still sounds like a robot wrote it.
You know the kind:
- "Leveraging synergistic solutions..."
- "Empowering dynamic innovation..."
- "Revolutionizing paradigm shifts..."
The problem isn't just about generating more content, it's about generating on-brand content.
Marketing teams are stuck between two frustrating options:
- AI that can produce content at scale but gets the details wrong
- Manual processes that are accurate but painfully slow
Enter retrieval-augmented generation (RAG).
RAG is how AI agents reference your brand's knowledge base. Unlike standard AI which relies on generic, limited training data, RAG-powered AI pulls from your actual brand guidelines, product listing details, content libraries, and customer behavior data.
This means content that's on-brand from the first draft, search that actually understands what customers want, and personalization that feels genuinely personal.
When AI agents miss the mark
A few marketing teams are using AI tools to churn out content at lightning speed, but here’s what often happens:
- Generic outputs: The content sounds robotic and impersonal, failing to resonate with your audience.
- Brand inconsistency: Without access to your brand guidelines, AI struggles to maintain tone, voice, and messaging.
- Inaccuracy and hallucinations: Generic AI tools are only as smart as what they’re trained on.
Imagine you’re a marketer tasked with creating product descriptions for a fashion brand launching a new line of clothing. If you’re not using an AI solution that employs RAG, you’re going to be stuck with generic information that’s completely irrelevant to the branding and messaging you are trying to convey to your users.
This is the content creator’s AI dilemma. But what if there was a better way?
Retrieval-Augmented Generation (RAG) differs from standard AI content generation, and here's how it matters:
- Intelligent search that gets it right: Product recommendations based on real purchase patterns, content suggestions that match the customer journey stage, and results that consider context and customer history
- Content creation: Creates on-brand content from the first draft, maintains consistent voice and reduces editing time.
- Personalization in motion: Personalized content paths that guide customers naturally
For example, when someone searches for "comfortable work shoes," they get actually comfortable, office-appropriate footwear and not just any shoes with "comfortable" in the description.
Master AI and supercharge your marketing
How RAG changes everything
Traditional AI models rely solely on pre-trained data. On the other hand, RAG dynamically retrieves relevant, real-time information, making AI-generated content more accurate and contextually relevant.
Image source: Optimizely
Think of Retrieval-Augmented Generation (RAG) as the marketing onboarding program for your AI agent. Here's what that really means:
1. Your AI finally gets your brand
Before RAG: "Let me check every single word this AI writes..."
After RAG: "This actually sounds like us – publish it!"
Your AI agent can now:
- Access your actual brand guidelines (not just guess at them)
- Learn from your successful campaigns (not generic marketing theory)
- Understand your real customer segments (not broad demographics)
- Know your product catalog inside out (no more outdated features)
2. It learns like a real team member
Unlike traditional AI, your RAG-powered assistant:
- Gets better with every piece of content it creates
- Adapts to feedback in real-time
- Maintains your brand voice across channels
- Stays current with your latest campaigns
3. It actually helps run campaigns
Your AI becomes a campaign partner that:
- Creates email sequences that understand customer journeys
- Generates social posts that nail your tone
- Suggests product recommendations that make sense
- Writes ad copy that reflects current promotions
4. It can make your website better
Your AI becomes a testing ally that:
- Spots website optimization opportunities from user data
- Creates test variants that match your brand voice
- Suggests targeting based on customer segments
- Analyzes results and recommends follow-up tests
- Maintains a backlog of data-backed test ideas
All of this happens automatically, letting your team focus on strategy instead of constant corrections.
Retrieval-Augmented Generation (RAG) use cases
Most businesses struggle with AI tools that work in isolation from their brand, creating more problems than solutions. RAG changes this by leveraging input or context from the customer's key artifacts or data, reducing editing time, and letting your team focus on strategy instead of fixes.
Image source: Optimizely
Here are a few examples of how RAG can improve marketing workflows while shaping the future of AI-powered teams:
1. Product recommendations
Turn your product content from generic to personalized and engaging:
- Pull specs directly from your product database
- Match your brand voice automatically
- Create personalized recommendations
- Get content approved on the first review
2. Intelligent email marketing
Improve your email campaigns with the following:
- Subject lines that drive higher open rates
- Content that references customer history
- Recommendations based on actual behavior
- Campaigns that adapt to engagement patterns
3. Search and discovery that delivers
Make your site search work better:
- Understand customer intent ("comfy work shoes" shows comfortable, professional footwear)
- Suggest products based on shopping patterns
- Return results from current inventory only
- Learn from every customer interaction
RAG can help your team finally focus on strategy while it helps you execute.
Making RAG work for your team
Before jumping in, assess your digital ecosystem:
- Which digital experiences consistently perform well?
- What customer segments drive the most value?
- Which products need the most content support?
This baseline understanding helps your RAG implementation focus on what matters most to your business.
Here's your playbook:
1. Gather your marketing intelligence
- Brand guidelines and voice documentation
- Successful campaign examples
- Customer segments and insights
- Top-performing content
- Product information that matters
2. Start smart
- Choose one content type you create often
- Train your AI agent with your best examples
- Test with different team members
- Scale what works and repurpose with AI
3. Track what matters
- Content approval rates
- Team time savings
- Campaign performance
- Brand consistency scores
What's next?
Your marketing team deserves better than an AI that creates more work. They deserve an AI that gets it right the first time. One that knows your brand, understands your customers, and makes everyone's job easier (and more enjoyable).
That's what RAG delivers. With Retrieval-Augmented Generation:
- Your teams spend more time on strategy and less on corrections
- Your content maintains quality even as you scale
- Your customers get experiences that feel personal
Want to learn more about RAG? See how to give your team an AI agent that actually helps.