A/B Testing: How to start running perfect experiments
The bigger a company is and the more senior folks are involved, the more people hesitate to experiment. To build a culture of experimentation, you need to rethink your approach. In this article, see more about the science of running perfect experiments and what kind of A/B tests can help you deliver conclusive test results.

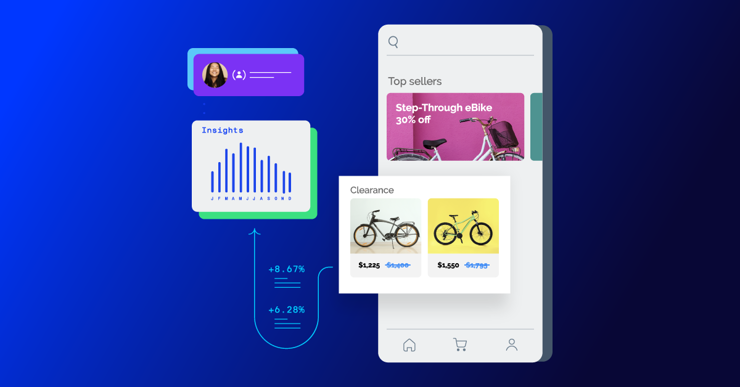
When creating digital experiences, we often believe we know what's best for our users. We redesign websites, tweak interfaces, and craft marketing messages with confidence.
But here's a sobering reality check: Only 12% of experiments actually win.
This statistic isn't just surprising—it's a wake-up call. It reveals a fundamental misunderstanding about how users interact with digital products and services. The truth is, that we're using reason and logic to understand something that is neither rational nor logical: human behavior.
Most user interactions are driven by subconscious processes, not the conscious, rational thinking we imagine. When we rely solely on our intuition or experience, we're often projecting our own biases rather than understanding our diverse user base.
This is where A/B testing and experimentation become invaluable. They're not just a tool for optimization—they're a window into the true nature of user behavior. By setting up controlled experiments, we can move beyond assumptions and let real-world data guide our decisions.
In this article, see how to challenge assumptions, make data-informed decisions, and build a culture of experimentation that embraces uncertainty and learns from failure.
The value of experimentation and leadership's role
Building a culture of experimentation brings tremendous value to companies. Harvard Business School did a study where they looked at the value testing provided to startups, especially in the ecommerce industry. They found that investors were willing to invest 10 % more dollars into companies that were experimenting than those that weren't.
Why experimentation matters:
- Risk mitigation: You can reduce costly mistakes by testing an idea first instead of building it into a full-fledged experience, product, or feature.
- Continuous improvement: Through continuous optimization, you can improve what’s already working on your website.
- Customer-centricity: You can align with actual customer preferences and deliver them personalized experiences they actually want.
- Competitive advantage: You can outpace competitors in meeting market demands.
And if you're worried about negative intervention from the leadership team, then the highest-paid person's opinion shouldn't always count most. Instead, if you’re a leader, you should:
- Encourage participation: Empower everyone to contribute ideas and run experiments.
- Embrace failure: Recognize failed experiments as learning opportunities.
- Lead by example: Challenge your own assumptions through testing.
Designing impactful experiments
Many organizations fall into the trap of over-analyzing and under-experimenting, making minimal changes out of caution. This approach:
- Takes too long to yield meaningful results
- Often produces effects too small to be significant
- Fails to keep pace with changing consumer behavior
Instead, embrace bold experimentation:
- Make larger changes: Test substantial modifications.
- Combine multiple elements: Test comprehensive redesigns rather than isolated tweaks.
- Prioritize impact: Focus on experiments with the potential for significant metric improvements.
In fact, in our lessons learned from 127,000 experiments, we found that experiments with larger changes and more than 3 variations saw 10% more impact.
Understand the maturity level of your program
To understand where your organization stands and how to progress in your experimentation journey, consider the following Experimentation Maturity Model:
1. Ad-hoc testing
Sporadic tests, no formal process, limited buy-in.
- Challenges: Inconsistent results, lack of resources
- Next steps: Establish a regular testing schedule, secure executive sponsorship
2. Structured experimentation
Dedicated testing team, defined processes, regular tests
- Challenges: Siloed information, limited cross-functional collaboration
- Next steps: Implement a centralized knowledge base, encourage cross-team experimentation
3. Data-driven culture
Testing integrated into all major decisions, cross-functional collaboration
- Challenges: Balancing speed and rigor, prioritizing experiments
- Next steps: Develop advanced prioritization frameworks, invest in faster testing infrastructure
Revolutionize your digital strategy
4. Predictive optimization
AI-driven testing, automated personalization, predictive modeling
- Challenges: Ethical considerations, maintaining human oversight
- Next steps: Establish ethical guidelines, continually reassess and refine AI models
The science of a good experiment
Let's say your company decides to add new filters to the product pages as a new feature. An engineer goes out, builds the code to create a filter, and gets ready to implement it on the top of the page. There’s only a single version of that filter. If it fails, we don't know if visitors don't want filters or if the usability of that filter is just poor.
Therefore, great if you want to have a filter, but have different versions of it. You can try it on the top of the page, on the left-hand side, and in other places. You can have it fixed or floating, and even change the order of the filters as well.
The benefit of this experiment is that once you've run this test, let’s say all variants of your filter lose. Now you know conclusively filters are not necessary for your customers. It is time to focus on something else. Or if a version of filters you tried wins, you only implement that quickly. Simply running one filter without any alternatives can lead to misinterpretation of results.
To get the most value from multivariate testing, approach it in a structured and systematic way. It involves:
- Defining a hypothesis: Before you start experimenting, have a clear idea of what you're testing, your target audience, and what you hope to achieve. Define a hypothesis in your template - a statement that describes what you expect to happen as a result of your experiment.
- Designing the experiment: Once you have a hypothesis, you need to design an experiment that will test it. It involves identifying the variables you'll be testing, calculating sample size, and determining how to measure the results.
- Running the experiment: With the experiment designed, it's time to run it. This involves implementing the changes you're testing and collecting quantitative data.
- Analyzing the results: Once the experiment is complete, it's time to analyze the A/B test results. This involves looking at the data you've collected and determining whether your testing hypothesis was supported or not.
- Iterating and learning: Use what you've learned from the experiment to iterate and improve your approach. It means using the data to make informed decisions about what to do next and continuing to experiment and learn as you go.
A/B testing ideas
Here are a few examples of ideas you can test.
- E-commerce: Test layouts, image sizes, and "add to cart" button placements. Experiment with single-page vs. multi-step checkouts. For example, Quip increased the order conversion rate by 4.7% by testing on the product display pages.
- Finance: Refine risk assessment models and test segmentation strategies for product recommendations. For example, RAKBANK improved website engagement by 37%.
- Media: Test recommendation algorithms. Experiment with paywall triggers and messaging. For example, Channel 4 launched 150+ high-impact tests in a year.
And if you want more ideas, we have a list of 101 ideas to help you optimize your digital experiences end-to-end.
How to start your experimentation journey
Data is critical for measuring the impact of your experiments and making data-driven decisions. It's important to have a clear understanding of the metrics you're using to evaluate success and to measure everything you can to get the most value from your experiments.
Firstly, let’s see what to avoid. When most people start with experimentation, they assume it is about making a simple tweak.
For example, if we the change color from red to blue, this will psychologically trigger the number of visitors to purchase more and increase the conversion rate. The beauty of a button color test is if it wins, you make money, and if it loses, you lose maybe 15 minutes of your time. It's very easy to run.
But to have a meaningful effect on user behavior, you need to do something very fundamental that's going to affect their experience and deliver a significant result.
For most businesses, experimentation is often very much on the periphery of the decision-making, so it's somebody who's just there to pick the coat of paint on a car that's already been fully designed and assembled. Or it is something driven by senior leadership. VPs and C-level executives are making all the calls, and there's a team on the ground that's merely forced to act out what they're asking for, but then they have the freedom to experiment.
Great experimentation is a marriage of all of these. A place where people have the right to make tweaks. They have the right to be involved in the design of the vehicle itself, and they are a partner to senior leaders in that decision-making process. Senior leaders come with great ideas, and they're allowed to augment them. They're not merely there to execute and measure the ideas of others.
Follow these steps to get going:
- Start small: Don't try to change everything at once. Instead, start with small experiments that can help you learn and build momentum in real-time.
- Focus on the customer: Experimentation functionality should be focused on delivering value to the customer. Make sure you're testing ideas that will have a real impact on their experience.
- Measure everything: To get enough data and value from your experiments, it's important to measure everything you can. This means tracking not just the outcomes, but also the process and the baseline metrics you're using to evaluate success.
- Create a culture of experimentation: Finally, it's important to have an A/B testing tool that uplifts the state of experimentation and innovation. This means giving people the freedom to try new things, rewarding risk-taking, and celebrating successes (and failures) along the way.
Wrapping up...
A testing program is a critical tool for driving digital transformation and Conversion Rate Optimization (CRO). By building a culture of experimentation, organizations can learn what works and what doesn't, and use that knowledge to drive change and deliver a top-notch user experience to their customers.
For a step-by-step guide to digital experimentation, check out the Big book of experimentation. It includes 40+ industry specific use cases of businesses that ran perfect experiments.